When Sipeed introduced MAIX-II Dock AIoT vision development kit, they asked help from the community to help reverse-engineer Allwinner V831‘s NPU in order to make an open-source AI toolchain based on NCNN.
Sipeed already had decoded the NPU registers, and Jasbir offered help for the next step and received a free sample board to try it out. Good progress has been made and it’s now possible to detect objects like a boat using cifar10 object recognition sample.
Allwinner V831’s NPU is based on a customized implementation of NVIDIA Deep Learning Accelerator (NVDLA) open-source architecture, something that Allwinner (through Sipeed) asked us to remove from the initial announcement, and after reverse-engineering work, Jasbir determined the following key finding:
- The NPU clock defaults to 400 MHz, but can be set between 100 and 1200 MHz
- NPU is implemented with nv_small configuration (NV Small Model), and relies on shared system memory for all data operations.
- int8 and int16 are supported with int8 preferred for speed and limited on-board memory (64Mb)
- 64 MACs (Atomic-C * Atomic-K)
- Memory-mapped register programmable from userspace
- Physical address locations are required when referencing weights & input/output data locations, meaning kernel memory needs to be allocated and the physical addresses retrieved if accessed from userspace.
- NPU weights and input/output data follow a similar layout to the NVDLA private formats, so formats like nhwc or nchw must be transformed before being fed to the NPU.
Those findings allowed him to adapt the code for the cifar10 demo from Arm’s CMSIS_5 NN library, removing all Allwinner closed-source binaries in the process. You’ll find the source code on v831-npu repository on Github, and can check out Jasbir post to find out how to try it out provided you have an Allwinner V831 board on hand.
The current code supports direct convolutions, bias addition, relu/prelu, element wise operations, and max/average pooling, and there’s more work to be done including the development of a weight and input/output data conversion utility and integrating into an existing AI framework.
The good news is the work should also benefit other platform features an NVDLA based AI accelerator including Beagle V SBC that has just started to find its way into the hands of developers in the few days.
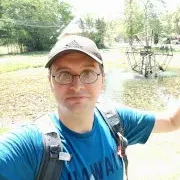
Jean-Luc started CNX Software in 2010 as a part-time endeavor, before quitting his job as a software engineering manager, and starting to write daily news, and reviews full time later in 2011.
Support CNX Software! Donate via cryptocurrencies, become a Patron on Patreon, or purchase goods on Amazon or Aliexpress
“Memory-mapped register programmable from userspace” and “Physical address locations are required” sounds pretty horrible from a security point of view :/
Sounds like almost any DMA hardware..
Sure, but you normally don’t talk directly to other DMA capable hardware from user space
Allwinner wants to keep this chip very secret. So I will help them out by not learning how to use it and by not selling any in my products. The best way to ensure secrecy is not having any customers — because having customers might expose those secrets!
BTW, we are happily progressing along using the RV1109/1126.
Is there a URL on the current status or blog for the RV1109/1126 Sipeed board?
We have boards from Firefly and two other vendors that are local to Shenzhen. I don’t think Sipeed has shipped yet.
The RV1109/26 is using the same platform as all of the other Rockchip CPUs. So you can use RK3399PRO to develop AI vision software and then port it over to RV1109 without much hassle. Just note RV1109 much slower than RK3399PRO.
Firefly has all of the code here:
https://gitlab.com/firefly-linux
Just use the RV1109 manifest to get the right build.
I would like to know why you claim that. Allwinner is a chinese brand like rockchip.. yeah, they offer quite bad products.. but I think this news is a gopd thing… not otherwise. Why you claim that buy allwinner is so harmful?
Allwinner is keeping the V831 SDK tightly controlled and so far I have been unable to get a copy out of them. I suppose I could pay Sochip $800 to get access. I’m just not very excited about paying $800 just to evaluate a chip that I haven’t even decided if I want to use in a design yet. And the last time I paid Sochip $800 they just gave me a board and a copy of the SDK and then never responded to any of our questions. On the other hand I easily acquired the Rockchip RV1109 SDK. It… Read more »
Very nice. I hope this progresses like the FPGA reverse engineering has. If we get a toolchain that can target a bunch of cheap SoCs with NPUs things get really interesting I think.