Sophgo SG2380 is an upcoming 2.5 GHz 16-core RISC-V processor based on SiFive Performance P670 cores and also equipped with a 20 TOPS AI accelerator using SiFive Intelligence X280 and Sophgo TPU that will find its way into a $120 desktop-class mini-ITX motherboard in H2 2024.
The RISC-V processor also supports up to 64GB RAM, as well as UFS 3.2 and SATA 3.0 storage, comes with an Imagination GPU for 3D graphics and a VPU capable of 4Kp60 H.265, H.264, AV1, and VP9 video decoding, plenty of interfaces, and the system can manage locally deployed larger-scale LLMs like LLaMA-65B without the need for external NVIDIA or AMD accelerator cards.
Sophgo SG2380 RISC-V SoC
Sophgo SG2380 specifications:
- CPU
- 16-core SiFive P670 (RV64GCVH) 64-bit RISC-V processor @ up to 2.5GHz with RISC-V Vector v1.0, Vector Crypto
- Cluster configuration – 12x 2.5 GHz performance cores, 4x 1.6 GHz efficiency cores
- Full RISC-V RVA22 prole compliance
- GPU
- Imagination AXT-16-512 high-mid-performance 3D GPU with support for Vulkan 1.3, OpenGL 3.0, OpenGL ES 3.x/2.0/1.1; 0.5 TFLOPS, 16 Gpixels, and 2 TOPS
- 2D graphic engine
- Video Processing Unit (VPU)
- Up to 4Kp60 10-bit H.265/HEVC, 8-bit H.264/AVC, 8-/10-bit AV1, 8-/10-bit VP9
- No hardware video encoder
- AI accelerators
- 8-core SiFive Intelligence X280 with support for BF16 / FP16 / FP32 / FP64, INT8 up to INT64
- Sophgo TPU coprocessor through VCIX interface up to 20 TOPS @ INT8 compatible with OpenXLA/IREE
- Memory I/F
- Up to 64GB RAM through a 128-bit DDR interface
- Support for LPDDR4 and LPDDR4x 3733Mbps with in–line ECC
- Support for DDR4 UDIMM, SODIMM @ 3200Mbps (no ECC)
- Storage I/F
- Video Output
- eDP 1.2 up to 4Kp60
- DP 1.2 up to 4Kp60 (USB-C Alt mode)
- HDMI 2.0 up to 4Kp60 with CEC and eARC support
- MIPI DSI up to 2Kp60
- Support for dual video output up to 4Kp60
- Camera
- Sophgo AI ISP with dual pipe
- 6x 2-Lane / 4 + 4 x 2 Lane image sensor input
- Interfaces – MIPI CSI2, Sub LVDS, HiSPi
- 2x I2C dedicated to image sensor interface
- Up to 6x 2MP cameras
- Audio
- HD Audio codec
- 3x DMIC
- 3x I2S, 1 of them share pin with HD Audio
- 1x PCM
- Networking – Gigabit Ethernet (RGMII interface)
- USB
- 1x USB 3.2 Gen 1 (5 Gbps) with DP Alt Mode, Power Delivery capable
- 1x USB 3.2 Gen 1 (5 Gbps)
- 2x USB 2.0 interfaces
- PCIe – PCIe Gen3 with 8x+4x+2x+1x+1x Lanes
- Other peripheral interfaces
- 3x SDIO/SD3.0
- 2x CAN 2.0
- 4x UART without trac control function or 2x UART with trac control function
- 8x I2C, SMBUS supported
- SPI/eSPI with 4 CS
- LPC
- PWM
- Fan detect
- Security
- Hardware AES/DES/SHA256
- True Random Number Generator (TRNG)
- Secure key storage, secure boot,
- SiFive WorldGuard
- 32Kb OTP flash
- Power Management – DVFS and ACPI support
- TDP – 5 to 30 Watts
- Junction temperature – -0°C to +105°C
- Package – FCBGA
Sophgo lists a range of applications for its processor, with single board computers, desktop PCs, mini PCs, laptops, workstations, Chromebooks, Android-based tablets, and edge servers (NAS or SMB server).
Oasis mini-ITX motherboard
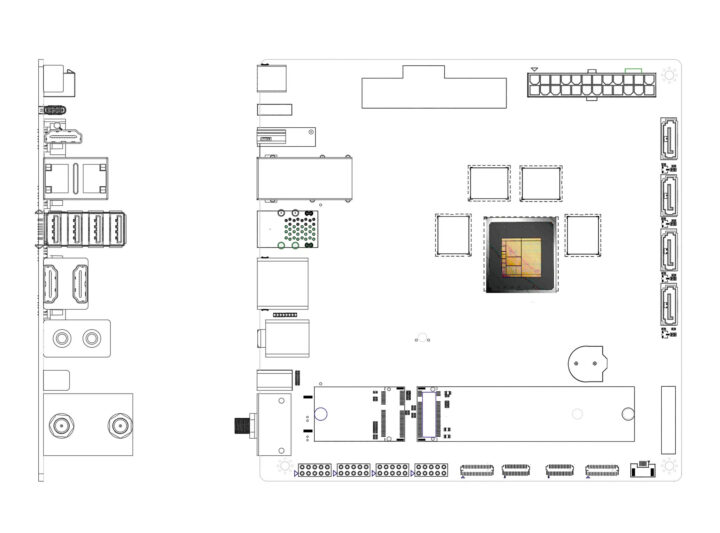
While the SG2380 SoC will be used for various applications, I expect a range of computers, mini PCs, and laptops considering the geopolitical situation between the US and China, the first platform based on the 16-core RISC-V processor will be the Oasis motherboard.
Oasis specifications:
- SoC – Sophgo SG2380 16-core RISC-V SoC as described above
- System Memory – Up to 64GB 128-bit LPDDR5 5500MT/s
- Storage
- Pluggable UFS module
- microSD card for OS booting and data
- M.2 M-Key socket for NVMe SSD (PCIe 3.0 x4)
- 4x SATA ports for HDD and/or SSD
- Video Output
- 2x HDMI ports up to 4Kp60
- 1x eDP with touch panel
- 2x MIPI DSI connectors
- Camera I/F – 2x MIPI CSI connectors
- Networking
- 2x 2.5GbE RJ45 ports
- 1x M.2 B-Key for 4G / 5G cellular module
- USB – 2x USB 3.0 ports, 2x USB 2.0 ports, 2x front panel USB 2.0 interfaces, 1x USB-C port with DP alt mode
- Expansion
- PCIe x16 slot with PCIe Gen 3 x8 signal
- 8x DIO, 2x CAN Bus
- Dimensions – 17 x 17cm (Mini-ITX form factor)
As you can see from the lack of photos of the board, development is still at the early stage, and the processor and motherboard are only expected in about 9 months (Q3/H2 2024), so I’d assume things may still change a bit, especially for the board.
But if you feel somewhat adventurous, you can already pre-order your Oasis motherboard from Arace for just $0.10 which will give you a coupon code for a 20% discount, or a starting price of starting $120. A few more details may also be found in the announcement.
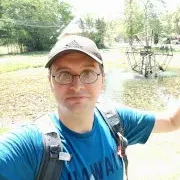
Jean-Luc started CNX Software in 2010 as a part-time endeavor, before quitting his job as a software engineering manager, and starting to write daily news, and reviews full time later in 2011.
Support CNX Software! Donate via cryptocurrencies, become a Patron on Patreon, or purchase goods on Amazon or Aliexpress
The board looks pretty good. Would make a nice NAS. I wonder which hd audio they are using and if that will have linux drivers.
It says 5-30w, i wonder if they mean 5w when idle. 30w is quite a lot, more than many would like for a nas.
16-core RISC-V SoC with TPU up to 20 TOPS make a nice Nas?
It looks an ML monster but how and what does https://opensource.googleblog.com/2023/03/openxla-is-ready-to-accelerate-and-simplify-ml-development.html do…
Yeah, have NAS always tiptoed up to 20 TOPS LLM? Maybe you’re upscaling your ColecoVision and Pippin speedruns through an AI upscaling texturing, but also deepdreaming a Shrek continuity? Also you use 10 64bit cores for stuff involving 6 cameras? But not h.265 encode. Bit of a media completist, there. GMI goes canvassing?
16 cores + gpu, 30 watt is not very much, as a nas it would use 5w i think.
Now that’s starting to be interesting with 12×2.5GHz cores. I’m confused about the DRAM though, the SoC supports DDR4/LPDDR4 and the mobo uses LPDDR5. We’ll see when it’s available.
[ seems specifications have variations, like that roadmap diagram showing PCI-e4&5;
not quite sure (prob. RAM, comparable to ZEN4 DDR5-6000 ~(top)96GB/s ) about that Bandwidth value bgThan 100GB/s, either ]
Make a might fine ZFS NAS, if it is available with ECC RAM.
M.2 M-Key socket for NVMe SSD (PCIe 3.0 x4) (3.938 GB/s)
4x SATA ports for HDD and/or SSD (2.4GB/sec)
Wìth a M.2 NVME SATA 3.0 Card 6 Ports (ASM1166) that could be up to 10 hard disks, configured as RaidZ3 10 (or RaidZ2 10) which would limit the read performance to that of a single hard disk (but that could still be more that enough to fully saturate both network links).
> ZFS NAS, if it is available with ECC RAM.
It’s almost 2024 and people still spread this silly urban myth about ZFS ‘needing’ ECC RAM 🙁
https://jrs-s.net/2015/02/03/will-zfs-and-non-ecc-ram-kill-your-data/
Maybe the third time will actually appear!
You never need “ECC” RAM, ever, but … (there is always a but), if you wish to measure bit flips, then you want ECC RAM. One fun example I particularly love is the higher rate of bit flips in the vicinity of cattle: https://www.jakepoz.com/debugging-behind-the-iron-curtain/
One thing to keep in mind for spinning rust and SSD that all data is stored using some form of LDPC (low density parity check) because the assumption is that every single read at the lowest level inside the device will be corrupt. Data leaving through external interfaces has been rebuilt from the corrupt low level raw data. In almost all storage some form of ECC is used. It is also used in CPU registers, L1, L2 and L3 caches.
Your other comments were in the moderation queue.
*ah* I guess I posted too much text and external links to trigger moderation. I’ll try and keep my replies shorter going forward.
You can skip the queue by omitting http(s) and www from links. Your comment posts immediately and a user can still select the text and open it with a context menu.
cnx-software.com/2023/10/20/microsd-express-memory-cards-2gb-s-speed/
Long replies are fine, otherwise tkaiser and myself would spend our time in the queue 🙂 I think it’s external links that trigger it mostly, as it happened to me when sharing product links already (that’s understandable to avoid spam).
I think this confusion emerged from all people wanting to build their NAS using ZFS and ECC, making some thinking that ZFS requires ECC. That’s not true, any FS is better protected with ECC, as it’s not fun to discover that some data were corrupted in memory after being read and checksummed, or before being checksummed and written. This FS might even check a bit more than other ones but this will not catch the classical memory corruption stuff caused by low-cost DRAM sticks or overheating RAM chips. When ECC is supported and available, for a NAS it remains a good idea. If not available, just do not overclock your RAM and use high-quality ones instead of super-fast ones.
> I think this confusion emerged from…
a former FreeNAS forum moderator who invented the ‘scrub of death’ myth: https://www.truenas.com/community/threads/ecc-vs-non-ecc-ram-and-zfs.15449/
From a support point of view though insisting on ECC RAM made a lot of sense since forcing FreeNAS users to buy expensive ‘server grade’ mainboards instead of using consumer grade crap.
As usual, people advocating full-throttle in either direction are always causing pain. I’d say that it’s always preferable to use ECC for storage, at least to avoid worrying, and there’s no reason one FS would be more or less sensitive to bit flips than others since they can happen anywhere in the chain.
This almost suggests that the SG2380 will become available in 2026 ?
https://forum.sophgo.com/uploads/default/original/1X/ae96e85d6637407eeaa8c9517a170bfe615d3370.jpeg
The above image is from the link below about the presentations planned for 2023-11-13 in the Denver Convention Centre.
https://forum.sophgo.com/t/sg2380-will-shine-brilliantly-on-second-international-workshop-on-risc-v-for-hpc/371
Thanks for you interest! I am Sandor, who wrote this slide, and this image means that we will release 2 different chips in 2024, SG2380 and SG2044.
SC2380 will tape out in May 2024, and silicon bring up in Sep 2024. If anyone interested in the first batch Oasis board in Sep, I suggest to reserve a coupon code.
I am very excited to reserve myself one of the first batch of Oasis board next year but in the meantime, when can we expect to see the Milk-V Meles released as its been ‘out of stock’ on Arace store for a while now with no recent updates on the Milk-V webpage…?
Milk, you’re a brave man putting a tape out date in stone on a chip that complex. Care for a bet….?
With the way that modern silicon works, you either bypass blocks that do not function 100%, or develop software workarounds. A product will be shipped. There is a reason for buying previously used, tested and debugged off the shelf silicon IP blocks for SPI/UART/CPU/PCIe/eMMC/USB/GMAC/…. it is all to lower the risks involved.
In Europe to acquire the pre-order coupon appears to be ninety five cents.
confirmed. I pre-ordered one anyway, it’s reasonable.
After reading more I would have to agree.
From: https://forum.sophgo.com/t/about-the-sg2380-oasis-category/359?u=sandor
Memory16G / 32G / 64G LPDDR5@6400Mbps, ECC, 128bit
Price120 US Dollars – 200 US Dollars
(which reading through the thread is after the 20% discount, I think)
If that is true, then the 4 different discounts possible in ~10 months time would be:
Coupon(20% discount), so ~$120 to ~$200
Super Early Bird(15%), so ~$127.5 to ~$212.5
Early Bird(10%), so ~$135 to ~$225
Kickstarter/CrowdSuppy Special(5%) so ~$142.5 to ~$237.5
no discount ~$150 to ~$250
I considered that I wouldn’t lose much at $1 especially if that helps them gauge market interest.
plus mail address of those interested
You forgot to mention the bank card, telephone number and home address.
bank card ? huh ?
In the end, could $120-$200 become €1140-€1900 for Europeans?
I think you exaggerate, but often towards to the EU it’s somehow the double price. Annoying
I can not argue with your logic where $0.10 becomes €0.95 for people living in Europe.
WOW! I GUESS 4x-5x powerful than RK3588s but cheaper
[ impressive, yes and if in need for special demand on (integrated) GPU (VPU) one should compare capabilities more in detail, maybe it’s just about waiting for determined/set specifications ]
Not cheaper as you have to add ram, but hell yeah I have ordered a coupon as its more Mac Mini than RK3588.
12x 2.5 GHz performance cores, 4x 1.6 GHz efficiency cores that are supposedly Cortex-A78 like in perf.
But its the Sophgo TPU coprocessor 20 TOPS @ INT8 that would seem at a glance similar to Apples AMX co-processor.
Then you get 8-core SiFive Intelligence X280 NPU.
The GPU is sort of OK Imagination AXT-16-512 GPU 0.5 TFLOPS, 16 Gpixels, and 2 TOPS.
The expensive bit is loading this up with ram as from the forum its DDR4/5 http://forum.sophgo.com/t/about-the-sg2380-oasis-category/359/7?u=sandor
The really exciting bit is what they tacked onto the end “the system can manage locally deployed larger-scale LLMs like LLaMA-65B without the need for external NVIDIA or AMD accelerator cards”
As yeah with 64Gb of DDR5-6400 is going to be about £200 and a NVME that unlike Apples soldered in we can upgrade and change at any time.
Its definately more Mac M1/M2 than RK3588 and a upgradeable without apples forced obselence.
Likely it has a strong chance to make it into ML server farms with the generous amount of Sata ports (Large datasets), but equally hits at a very attractive price of a home server, running your own private LLM as a Home Assistant.
@Sandor(xintong) do you guys have plans on providing optimised LLMs for the X280/Sophgo TPU coprocessor and frameworks?
[ is ~10GB sufficient for storage of a LLaMA-65B model file? (Thx) ]
I go off what they are doing at LLama.cpp as they are doing great stuff there.
Prob not https://github.com/ggerganov/llama.cpp#memorydisk-requirements which is a bit outdated but good enough for rule of thumb.
Its all about how much quantisation and effect on perplexity and context windows you use.
Also though loading up with a load of ram could also make this a very cost effective training platform as I may be wrong about this but you could stack the devices but need to keep in a single ram instance.
64gb for training many models is super usefull and vastly cheaper than any GPU with dedicated…
For running though its definately enough if quantised.
Model Original size Quantized size (4-bit)
7B 13 GB 3.9 GB
13B 24 GB 7.8 GB
30B 60 GB 19.5 GB
65B 120 GB 38.5 GB
Likely with Zram and some tweaks you could squeeze in a 8bit quant 65B param model in 64Gb
Its how fast an optimised framework for the Sophgo SG2380 could provide.
If you look how Apple optimisation and results they provide a Mac Mini/Pro to stupid things like the Ultra make amazing Gflops/Watts LLM servers and not expecting the same for $120+Ram but if optimised I am presuming performance will be considerable as the hardware focus is very much ML and they name drop ‘LLaMA-65B without the need for external NVIDIA or AMD accelerator cards’ which actually at this price range is awesome.
https://www.amazon.co.uk/Corsair-VENGEANCE-Regulation-Heatspreader-CMK64GX5M2B5600C40/dp/B09WN2H42Y
£158.47
I did reply but for some reason deleted have a look at https://github.com/ggerganov/llama.cpp#memorydisk-requirements
Doh its back again … 🙂 And now gone again
If this thing does things at my guesstimate speed then + ram its quite a bargain.
[ (Thx), IIUC that’s an example for 64GB ram requirement? and maybe there’s a file compression advantage for the model files with a 12x(/4x) -ge ~A78 cores?
Is a x280&TPU(‘contain thousands of multiply-accumulators that are directly connected to each other to form a large physical matrix’)&VCIX system capable of OpenCL(‘They do not need to learn CUDA and OpenCL like GPUs, but can use standard compiler solutions such as Pragma and MPI.’)? ]
Usually you want to use a Q5K_M model that provides quality slightly above Q5_1 in a size between Q4_1 and Q5_0. It generally gives good trade-offs. Very roughly speaking you’ll need bits/8*model size + approx 10% of it for the context. So a 65B model at Q5K_M could be around 44G in operation, so it might just tightly fit in 48G RAM. In any case, it’s also interesting to consider more recent variations of these models, particularly those mixed with orca and platypus. Mistral (and its fork dolphin-2.1-mistral) is surpassing everything at only 7B but only exists at 7B, so while it does have an excellent understanding of the language and accurate instruction following, it doesn’t know much of the world compared to llama-65b and is quite prone to hallucinations.
What matters in the end is that the accelerator on this board becomes supported by llama.cpp when the board reaches stores so that we can all make use of it with any of the thousands models available depending on our respective use cases.
Yeah it would be great if it was, I msg spammed Georgi on twitter in a vain attempt. 🙂
Even multiple domain models in a form of home chatGPT…
I think its all geared up for MLIR https://github.com/openxla Jax …
Dunno I just steal from the shoulders of giants on github and play. I keep thinking though if sucessfully supported that is exactly on the button where my pocket could not extend to with a Mac Mini with large ram.
In fact I think the Mac Mini is great but I hate how forced obsolence of the NVME & Ram via soldered in which this solves.
I have my preorder voucher ordered and when avail I will take the leap as this thing is truly fascinating and fills a niche that is exactly something I have been searching for.
RK3588(s) is a great little Soc but for a cutting edge Home AI / Voice Server it falls short but the above…
I am still confused about the DDR4/5 support though as what is that about, but hopefully DDR5 as with GPUs mem bandwidth with LLMs is a huge bonus.
[ (Thx), me reading there are ‘mixed-precision optimizer’ ((b)float16-mixed can be comparable to (b)float32’s prediction accuracy), what might improve precision, while reducing model size for training/inference. There was a number being ~1TB of data input for ~current LLMs. Probably, there’s lower expectation for reduced model sizes with demand for precision also, thus being ~10’s of GB ram for inference tasks. Another possibility seems sharing training/requests over several GPUs or TPUs?
training for 16bit(FP,BF) optimizers ‘trillion-parameter model with an optimizer in 16-bit precision would ordinarily require around 16 terabytes (TB) of memory to accommodate the optimizer states, gradients, and parameters’
with increasing model sizes and ML content available, there could be increasing ML content compromising from a www automatically retrieved model input data(?), thus ML/AI is about quality input and/or human (controlled) feedback structures/methods, also(?)
one example for a ML/DL model data base, e.g. ‘www.openml.org’ ]
Dunno I have an interest in Smart Speaker Assistants but my Rtx3050 8gb is pretty woeful and steals my main desktop.
I am thinking I could load that up with 64gb and just leave it ticking away if I can get it to support various frameworks.
I noticed Int8 speed was the same as 16bit which I find generally Int8 on the Neon optimised RK3588 is usually a sweet spot.
There is so much unknown about this thing that really purchase is an act of faith but for $100 its such an intriguing piece of kit that in 10 months time its worth a punt.
If rockchip are watching a Cortex-X SoC would also be sweet 🙂
I’ve been waiting for a company to release a desktop-class motherboard for a reasonable price that use the Sifive higher performance codes. And unlike the VisionFive2 this has a riscv64 processor that supports the hypervisor extension, along with enough ram and cores to make it a wonderful VM platform for testing, etc. I was having issues buying 1 coupon, kept telling me the payment method failed, so if i bumped up the price by buying 10 coupons, it finally went thru.
I’m having issues paying, Ali Pay, PayPal and Google Pay, they all fail, whether I try paying in my local currency or USD.
I am having the same problem. I can’t preorder. I have tried different payment methods and cards, all end up with order processing error
I had issues ordering too. I think there is a problem paying less than credit card processing fees. I bought 10 coupons and the sale went thru.
Thanks!
I ordered 10 coupons and managed to enter an order.
I emailed their support a few days ago and yesterday got a response from them acknowledging the issue.
They told me they increased the coupon price to 5$, but this will be deducted from the final payment.
This sounds great, but given current track records…
who is sophgo ? it came out of nowhere or what ?
https://www.sophon.ai/about-us/index.html its really been forced by the Chips act and China is doing its own thing…
Sophon(Beijing) is a subsidiary of Bitmain
It’s a relatively new company, but we wrote about their chips a few times already:
https://www.cnx-software.com/2023/08/02/firefly-aio-1684xq-motherboard-features-bm1684x-ai-soc-with-up-to-32-tops-for-video-analytics-computer-vision/
https://www.cnx-software.com/2023/06/30/64-core-risc-v-motherboard-and-workstation-enables-native-risc-v-development/
https://www.cnx-software.com/2023/03/31/sophon-bm1684-bm1684x-edge-ai-computer-32-tops-decodes-32-full-hd-videos-simultaneously/
This seem like it would be great, I will wait for it to actually come out though before ordering.
But it really has a lot of cores, a lot of accelerators and everything really. Though for some applications,